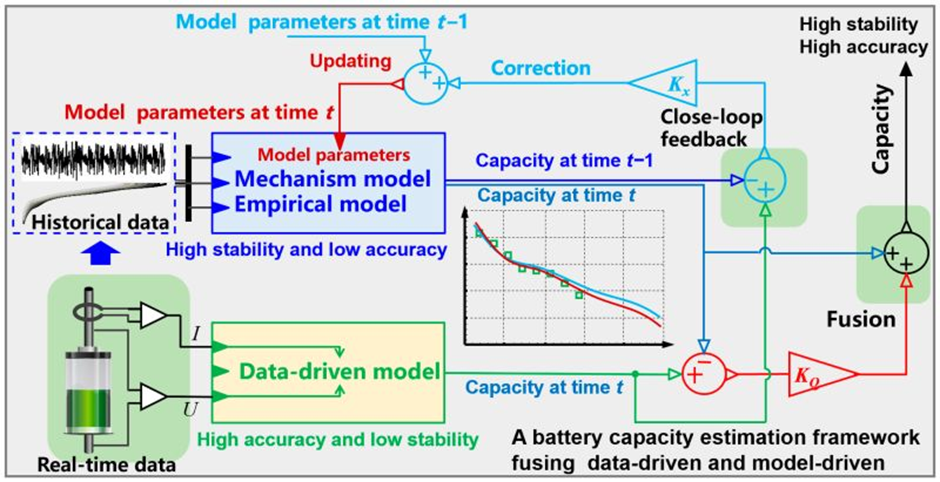
Estimating the battery state is a significant challenge in the 'Battery Management System (BMS)' due to the nonlinear characteristics of Li-ion batteries. The complicated process involves estimating the complex internal state of the battery with limited external signals & predicting the long-term state with short-term test signals.
Evolution of Battery Management Systems (BMS)-
1. Zero Management (1st Gen): Basic tasks such as voltage detection & controlling charge & discharge.
2. Simple Management (2nd Gen): Monitors battery data, including current, voltage, & temperature, from a few batteries. A simple control algorithm to prevent overcharge & over-discharge, enhancing battery safety & lifespan.
3. Advanced Management (3rd Gen): As the number of batteries increased, BMS became more intelligent & sophisticated. Started managing a larger number of batteries, with features like state estimation, fault diagnosis, thermal management, & fast charging. Faces challenges in extreme conditions due to the increasing energy density of batteries.
4. Intelligent Management (4th Gen): Next phase of BMS evolution focuses on long-term, accurate management of large-scale batteries under complex conditions. It adds advanced functions such as ultrafast charging, active safety control, & high-level interactions like vehicle-to-grid (V2G), vehicle-to-home/buildings (V2H/B), & vehicle-to-vehicle (V2V). Technologies like intelligent sensing, big data, AI algorithms, digital twins, & blockchain are integrated into this generation of BMS, enabling active, cooperative management & full life cycle control.
Key Challenges in Battery State Estimation & Proposed Solutions-
1. Online Extraction of Electrochemical Characteristics: Proposed solutions include,
- Developing high-precision, low-cost methods that can measure the internal state of batteries.
- Using advanced models to extract more information from limited external signals like current, voltage, & temperature.
2. Online Identification of Battery Aging Patterns: Possible solutions include,
- Developing methods that can go deeper into the battery to capture the essence of aging, thereby improving the accuracy of State of Health (SOH) estimation.
- Enhancing aging models to better account for long-term & hidden characteristics.
3. Accurate Prediction of Battery Life-cycle Aging Trajectory: Possible solutions include,
- Develop techniques that can accurately map & predict the entire life-cycle aging trajectory.
- Build models robust enough to handle the nonlinearity of battery aging & accurately predict hidden characteristics like the knee point effect.